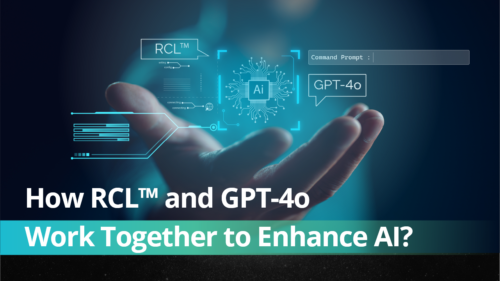
The field of artificial intelligence has been on the up in recent years. Recent developments are altering our understanding of natural language processing (NLP) and machine learning. OpenAI’s multimodal AI model, GPT-4o, is one such advancement that provides enhanced text generation, contextual awareness, and reasoning abilities.
On the other hand, Random Contrast Learning (RCL™), pioneered by Lumina AI, offers a fundamentally different approach to AI training. One that prioritizes efficiency, scalability, and cost-effectiveness.
Combining GPT-4o and RCL™ allows for faster, more intelligent models that don’t require a lot of processing power, which is a revolutionary change in AI development. However, what is the strength of this partnership?
Let us look at how GPT-4o and RCL™ collaborate to transform AI deployment and training across industries.
Understanding RCL™ & GPT-4o
The most recent version of OpenAI’s GPT models is GPT-4o. By providing more sophisticated language understanding, it offers several improvements over earlier iterations and is perfect for activities like chatbots, summarization, and detailed problem-solving.
However, RCL™ is a revolutionary approach to training AI models developed by Lumina AI. Instead of traditional deep learning techniques that require vast datasets and high-end GPUs, RCL™ optimizes training through contrast-based learning. The best thing is that RCL™ requires far fewer examples to achieve high accuracy, making it an excellent choice for industries where data collection is challenging.
How do RCL™ & GPT-4o Work in Combination?
Integrating GPT-4o with RCL™ addresses key challenges in AI by enabling seamless natural language interactions with specialized RCL-trained models.
In this integration:
- GPT-4o exclusively handles natural language interactions, translating user inputs and clearly presenting inference results.
- RCL™ independently manages specialized modeling tasks, performing inference solely based on models trained through RCL’s contrast-based methodology.
Crucially, RCL™ does not modify, fine-tune, or otherwise influence the training or internal workings of GPT-4o itself.
Faster Model Training & Adaptation
Training specialized AI models traditionally demands significant time and resources. RCL™’s contrast-based learning dramatically accelerates training. Combined with GPT-4o’s advanced natural language capabilities, this integration enables:
- Quick training and deployment of models without extensive GPU clusters.
- Easy adaptation and scalability across various domains and use cases.
Reduced Computational Costs
GPT-4o, while powerful, requires substantial resources at scale. RCL™’s optimization for CPU-based training and inference significantly reduces costs by allowing AI to run effectively on standard hardware, resulting in:
- Lower infrastructure investment requirements.
- Accessible AI deployment for startups, smaller businesses, and industries.
Enhanced Model Efficiency
Efficiency in AI is more than speed; it’s also about precision and minimal resource use. RCL™’s methodology enhances efficiency, enabling GPT-4o to:
- Reduce memory usage during inference.
- Improve performance for real-time applications such as image diagnostics and financial forecasting.
- Lower latency, enhancing responsiveness in chatbots and AI-driven interactions.
Increased Personalization in AI Models
GPT-4o excels at contextual understanding, while RCL™ enables rapid training on specialized datasets. This facilitates:
- Personalized AI assistants tailored to specific industries or needs.
- Improved accuracy in domain-specific tasks, such as medical diagnostics.
- Quick responsiveness to new data, trends, or localized information.
Sustainable AI Development
Energy efficiency is crucial in AI development. RCL™ optimizes GPT-4o’s inference processes, promoting:
- Lower energy consumption and a reduced carbon footprint in AI research.
- Environmentally conscious AI innovation through minimized resource use.
- Greater accessibility for smaller organizations and research institutions due to decreased operational costs.
Real World Scenarios of RCL™ X GPT-4o
The integration of GPT-4o and RCL™ has vast applications across industries, including:
- Healthcare:
By concentrating on weak patterns and making sure that models generalize well across unseen data, RCL™ can help increase model accuracy in outcome prediction and disease diagnosis. This is particularly helpful when dealing with heterogeneous medical data (such as rare diseases and diverse populations). Furthermore, RCL™ can help when patient data is sparse or unbalanced by lowering the quantity of data required to train models.
- Insurance:
RCL™ enhances risk profiling and forecasts by spotting subtle patterns in massive insurance datasets that conventional machine-learning methods could miss. Furthermore, it might be easily integrated with current machine learning pipelines for risk assessment and claims processing, improving performance with little data.
- Banking:
By enhancing generalization and adjusting to new fraud strategies without requiring a lot of training data, RCL™ can assist in identifying new fraudulent patterns. Banks can offer more precise and customized services with less data by incorporating RCL™’s capabilities into customization engines. This is particularly helpful for high-value clients or infrequent occurrences in transaction data.
The Future of AI with RCL™ x GPT-4o
The combination of RCL™ and GPT-4o defines a new age of AI development that is more accessible, affordable, and fast. Through the utilization of GPT-4o’s sophisticated generative capabilities and RCL™’s efficiency-first methodology, AI solutions that were previously unattainable owing to resource constraints could be created.