Simple, fast, accurate classification ai on cpu
Case Study: Applying RCL to Medical Imaging
RCLC is a derivative of Random Contrast Learning (LuminaRCL™) that specializes in classification. Lumina’s research is primarily concentrated in medical imaging, given RCLC’s ability to tackle classification problems of several shapes.
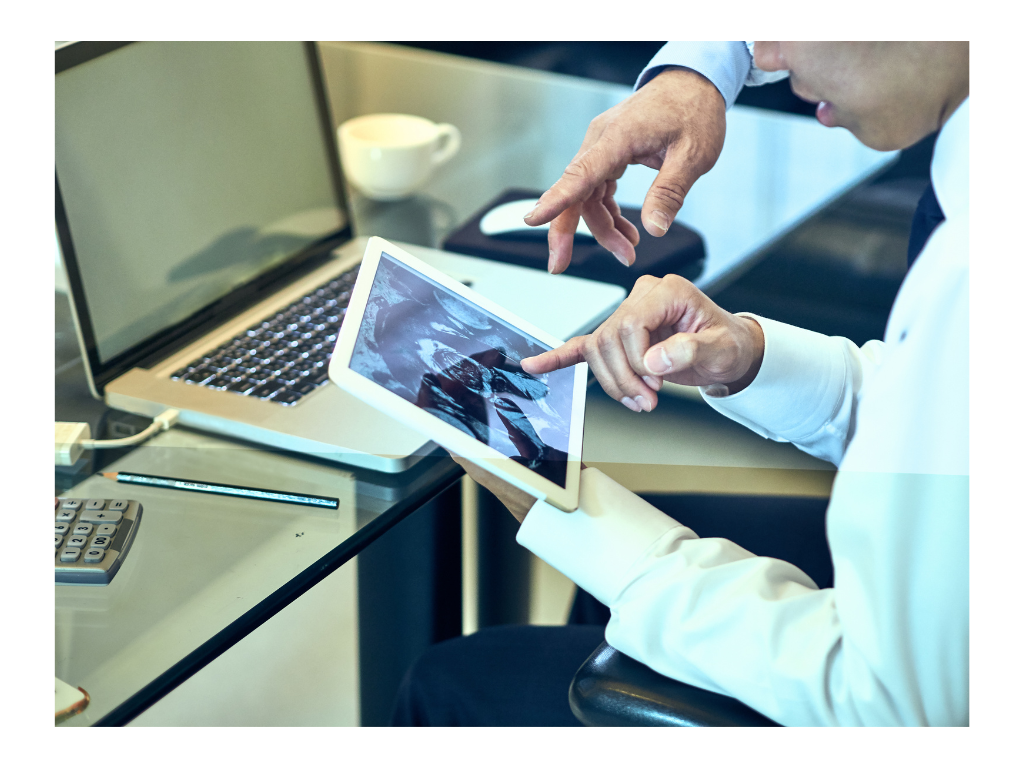
AI in Radiology
Radiology is well positioned for the application and adoption of artificial intelligence.
- Radiology has been significantly impacted in the post-COVID healthcare environment.
- AI adoption in radiology is increasing.
- Workloads in radiology have substantially increased in the last 20 years with the advent of PACS (Picture Archiving and Communications Systems), increased utilization of advanced cross sectional imaging with much larger sets of data to analyze, voice recognition software and self-editing, and an overall increase in the number of imaging studies read¹.
- Other factors contributing to a sense of work overload include long work days with increased after hours responsibilities, greater expectations for report turnaround times, conflicting demands on time (clinical, academic, administrative), and inadequate staffing¹.
- Nearly two-thirds (61%) of radiologists suffer from burnout, up from 36% in 2013 to 49% in 2017.
¹ Chetlen AL, Chan TL, Ballard DH, Frigini LA, Hildebrand A, Kim S, Brian JM, Krupinski EA, Ganeshan D. Addressing Burnout in Radiologists. Acad Radiol. 2019 Apr;26(4):526-533. doi: 10.1016/j.acra.2018.07.001. Epub 2018 Jul 31. PMID: 30711406; PMCID: PMC6530597.
- AI excels in analysis of images, with radiology having an established digital workflow and universal standards for image storage¹.
- Although the penetration of AI in the U.S. market is currently estimated to be only 2%, the readiness of radiologists and the potential of the technology indicate that further translation into clinical practice is likely to occur⁴
-
The FDA has approved 200+ commercial AI applications².
- 30% of radiologists use AI clinically,
- 70% have no plans to pay for AI,
- 80% of those not using AI see “no real benefit.³”
¹ LeCun Y, Bengio Y, Hinton G. Deep learning. Nature 2015;521:436-44.
² The Current and Future State of AI Interpretation of Medical Images Pranav Rajpurkar, Ph.D., and Matthew P. Lungren, M.D., M.P.H. https://www.nejm.org/doi/pdf/10.1056/NEJMra2301725?articleTools=true
³ “An Essential Roadmap for AI in Radiology” https://www.acr.org/Practice-Management-Quality-Informatics/ACR-Bulletin/Articles/September-2022/An-Essential-Roadmap-for-AI-in-Radiology
⁴ The Current and Future State of AI Interpretation of Medical Images Pranav Rajpurkar, Ph.D., and Matthew P. Lungren, M.D., M.P.H. https://www.nejm.org/doi/pdf/10.1056/NEJMra2301725?articleTools=true
Research
In recent testing, LuminaRCL™‘s classification algorithm was nearly perfect in its identification of malignant and benign tissue in the identification of breast cancer in biopsy images.
Recent Work
Lumina has applied RCLC to classify benign and malignant tissues in breast cancer biopsy images, lymph node biopsy images, and brain cancer MRI images.
building your medical imaging workflow
Apply RCLC via Lumina’s products and experience the future of classification.
Download PrismRCL™ on your Windows machines to train, iterate and combine models from a simple command line interface. Keep your data within your environment and easily enhance your classification workflows.
Try it free for 30-days while in Beta.
Train your models utilizing the LuminaRCL API for large workloads, on our hardware. Upload data, train your models, and iterate quickly. No minimum hardware specifications.
Unsure which tool is best for you?
Read our in-depth comparison of PrismRCL and the LuminaRCL API to determine which product is best for you & your datasets.