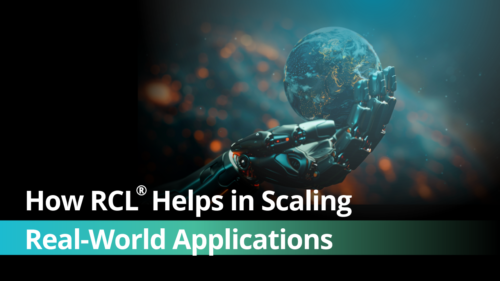
Real Life example of Artificial Intelligence shows it as a driving force for change, altering how businesses operate and transforming entire industries.
Despite AI’s enormous promise, several obstacles must be addressed before it can be effectively applied in the real world. For AI to have a significant influence, businesses across various industries, including banking, insurance, and healthcare, must effectively manage complex data environments, consider ethical concerns, and overcome computational limitations.
This is what RCL™ does. It reduces challenges to AI adoption by enhancing the way machine learning models handle and generalize data, thereby increasing the effectiveness, efficiency, and accessibility of advanced analytics across various industries.
Now, let’s explore the key challenges in scaling AI and how RCL™ is well-suited for more thoughtful and responsible AI deployment.
Redefining AI Usage in Healthcare
Persisting Challenges
- Disease Diagnosis & Prediction
It is still difficult to diagnose diseases like Cancer and heart disease early, mainly when algorithms are unable to handle complicated datasets like genomes and medical imaging.
- Accuracy in Medical Imaging
Diagnostic errors frequently result from AI models that analyze MRIs, CT images, and X-rays, failing to detect tiny patterns.
- Predicting Patient Outcomes
Forecasting patient responses to treatments requires integrating diverse data, including genetics, medical history, and real-time monitoring.
How can RCL™ play a role:
- Improved Generalization
In cases involving rare diseases or diverse patient populations, RCL™ improves models by identifying weak patterns, enabling more precise diagnosis and predictions.
- Enhanced Medical Imaging
RCL™ improves early detection rates by allowing AI to find anomalies in scans that conventional models might overlook through the improvement of classification capabilities.
- Effective Learning
RCL™ is especially helpful when patient data is limited or unbalanced, as it eliminates the need for large datasets and is more resistant to overfitting, ensuring trustworthy AI-driven insights with minimal computing overhead.
Strengthening AI Utilization in the Insurance Industry
Persisting Challenges
- Fraud Detection
Insurance fraud is becoming increasingly prevalent as scammers employ more sophisticated techniques.
- Risk Assessment
Based on behavioral data, it remains challenging to predict accurately which consumers are most likely to submit claims.
- Processing Claims and Pricing
AI models that can adapt to diverse consumer profiles and external variables are essential for determining optimal pricing and evaluating the severity of claims.
How can RCL™ play a role:
- Detecting Hidden Patterns
By spotting minute discrepancies in claims data, RCL™ improves fraud detection over traditional rule-based algorithms.
- Improved Precision in Risk Assessment
RCL™ enhances risk forecasts by identifying subtle clues in consumer behavior, guaranteeing accurate and equitable policy pricing.
- Smooth Integration
RCL™ effortlessly improves current AI-powered insurance processes, maximizing claims handling with the least amount of data while preserving effectiveness.
Supporting Banking & Financial Services with AI
Persisting Challenges
- Credit Scoring & Risk Assessment
Fair loan evaluations are challenging since AI models must assess creditworthiness using small or insufficient previous data.
- Fraud Prevention
Adaptive models that can keep up with changing attack techniques are necessary for detecting identity theft, card fraud, and money laundering.
- Customer Retention & Customization
Banks strive to offer personalized financial services but often lack sufficient high-quality data to train AI models.
How can RCL™ play a role:
- Enhanced Credit Rating
RCL™ helps identify hidden trends in borrower behavior, strengthening risk assessments even when data is sparse.
- Detecting Fraud Adaptively
Without requiring significant retraining, RCL™ helps financial institutions identify new fraud trends by enhancing generalization.
- Tailored Services Using Minimal Data
By enhancing AI personalization techniques, RCL™ enables banks to deliver customized financial solutions and foster enhanced customer interactions with reduced data requirements.
Scaling AI with RCL™: A Smarter, More Accessible Future
The ability of AI to grow while maintaining its effectiveness, ethics, and accessibility is essential to its success. Many industries find it challenging to deploy AI, as traditional machine learning models often require expensive technology and large datasets. RCL™ defies this standard by offering a scalable, more resource-efficient substitute.
It enables the adoption of AI in businesses that require it the most by processing weak patterns, improving generalization, and operating smoothly on standard CPUs. RCL™ enables companies to utilize AI without incurring undue computing expenses, whether that be for enhancing risk assessments, preventing fraud, or improving early disease diagnosis.
By emphasizing accessibility and efficiency, Lumina AI is setting the standard for the practical application of AI. Solutions like RCL™ will aim to guarantee that, as industries continue to drive AI innovation forward, AI stays not only potent but also useful and accessible to everybody.