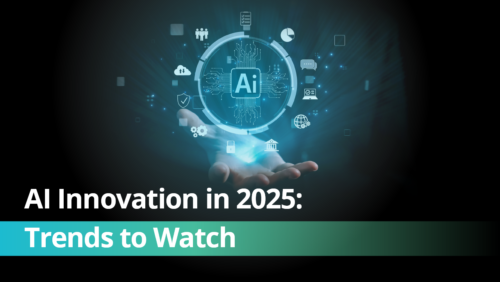
2025 marks a pivotal year in the evolution of artificial intelligence.
AI has evolved from an intriguing concept to a transformative force, powering significant advancements in sectors like healthcare and infrastructure optimization. By pioneering innovation in artificial intelligence, businesses and governmental organizations can increase productivity, improve decision-making, and enhance public services.
In this article, we’ll highlight the most significant AI trends emerging this year, offering insights into what they mean for technology leaders, practitioners, and those curious about the future of AI.
1. Rise of Agentic AI
Agentic AI refers to intelligent systems capable of independently making decisions and executing tasks without direct human oversight. These AI agents are capable of handling intricate workflows, adjusting to novel surroundings, and carrying out activities with little intervention. As companies look for more scalable and effective solutions, the adoption of this technology is accelerating.
Unlike their predecessors, these cutting-edge AI systems are specifically designed to support goal-oriented outcomes independently. These can range from business-specific objectives, such as boosting sales or supply chain optimization, to personal ambitions.
2. Emergence of Multimodal AI
Multimodal AI refers to machine learning models designed to seamlessly handle multiple types of data, such as text, images, audio, video, and even code within a single, integrated framework. Currently, most AI models and applications focus primarily on one or two data types, such as text or video, but multimodal approaches, capable of seamlessly handling multiple data types simultaneously, are rapidly becoming the new standard.
Multimodal approaches are critical because they more closely reflect real-world complexity, enabling AI systems to interpret context, enhance accuracy, and deliver richer, more versatile insights across diverse applications.
3. Lumina AI’s Random Contrast Learning® Algorithm
Random Contrast Learning (RCL®) is a proprietary algorithm developed by Lumina AI designed to optimize artificial intelligence training specifically for CPU hardware. This marks a significant departure from the current market, dominated by neural networks reliant on costly, resource-intensive GPU infrastructure. By streamlining AI model creation, RCL® significantly enhances speed, accuracy, and cost-effectiveness, making sophisticated AI capabilities accessible to a broader range of organizations.
This contrasting approach is critical because it addresses the financial and environmental limitations associated with conventional AI methods. Practical applications of RCL® span multiple sectors, as demonstrated by Lumina AI’s PrismRCL, which significantly reduces operational costs and energy consumption, ultimately improving sustainability and ROI in large-scale AI deployments.
Unlike traditional AI frameworks that require extensive computing power, RCL® is designed to be lightweight yet robust, capable of scaling effortlessly across industries without sacrificing performance. This enables organizations with limited IT infrastructure to leverage cutting-edge AI without undergoing massive overhauls or capital investment. It also shortens development cycles and accelerates time-to-insight, giving businesses a sharper competitive edge in fast-moving markets.
Furthermore, as data privacy regulations tighten and decentralized AI gains traction, RCL® offers a future-proof alternative. Its CPU-optimized architecture allows more AI tasks to be processed locally, reducing reliance on data transfers and minimizing risk, making it a strong contender for privacy-sensitive and mission-critical applications.
4. Increased Reliance on Synthetic Data
Synthetic data refers to data artificially generated by AI models, created to mimic real-world data for use in training and validating machine learning systems. Given the speed at which GenAI is developing, progressive companies rely upon it to generate vast volumes of data for ML model training. Synthetic data can be useful in many domains, including healthcare, insurance, and medicine, because it replicates the features of real-world data.
Synthetic data enables organizations such as banks and automotive manufacturers to develop safer, higher-quality products and services while ensuring complete data privacy and compliance with regulatory standards like GDPR and HIPAA.
5. Tech with Energy-efficiency & Spatial Computing
Energy consumption is soaring due to all these new developments in AI. Fortunately, the tech sector is committed to promoting sustainability. Greener technology without compromising performance will be the norm in 2025, from AI chips that consume electricity to data centers powered by renewable energy. The goal of energy efficiency goes beyond cost savings—it ensures our planet can sustainably support our ongoing technological progress.
Spatial computing fundamentally involves blending the digital and physical worlds, seamlessly integrating virtual experiences into real-world environments. Consider digital twins that replicate a factory’s operations or augmented reality spectacles that provide real-time directions while you walk. This technology is revolutionary.
Conclusion:
The AI environment in 2025 is characterized by notable developments. Companies like Lumina AI are at the forefront, creating cutting-edge solutions that not only improve AI performance but also address important problems like affordability, sustainability, and accessibility. Individuals and organizations hoping to leverage AI for future success must remain informed and flexible as these trends continue to change.